Imagine you’re learning different categories of fruit, but the emphasis on features changes depending on the order in which you study them. That’s where the Sequential Attention Theory Model (SAT-M) comes in! Unlike other models, SAT-M not only considers the category assignment of an item but also how it relates to its neighboring items. By studying data from experiments with different trial sequences (interleaved vs. blocked), researchers found that SAT-M accurately captures the effect of local context and predicts which training method will result in better testing performance. In fact, other models like ALCOVE and SUSTAIN, as well as a version of SAT-M without locally adaptive encoding, didn’t fit the results as well. And guess what? SAT-M even provided insights into learners’ behaviors during training. It’s fascinating to see how our brains adapt to changing contexts when learning new things! Curious to dive deeper? Check out the research article linked above!
Abstract
Although current exemplar models of category learning are flexible and can capture how different features are emphasized for different categories, they still lack the flexibility to adapt to local changes in category learning, such as the effect of different sequences of study. In this paper, we introduce a new model of category learning, the Sequential Attention Theory Model (SAT-M), in which the encoding of each presented item is influenced not only by its category assignment (global context) as in other exemplar models, but also by how its properties relate to the properties of temporally neighboring items (local context). By fitting SAT-M to data from experiments comparing category learning with different sequences of trials (interleaved vs. blocked), we demonstrate that SAT-M captures the effect of local context and predicts when interleaved or blocked training will result in better testing performance across three different studies. Comparatively, ALCOVE, SUSTAIN, and a version of SAT-M without locally adaptive encoding provided poor fits to the results. Moreover, we evaluated the direct prediction of the model that different sequences of training change what learners encode and determined that the best-fit encoding parameter values match learners’ looking times during training.
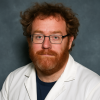
Dr. David Lowemann, M.Sc, Ph.D., is a co-founder of the Institute for the Future of Human Potential, where he leads the charge in pioneering Self-Enhancement Science for the Success of Society. With a keen interest in exploring the untapped potential of the human mind, Dr. Lowemann has dedicated his career to pushing the boundaries of human capabilities and understanding.
Armed with a Master of Science degree and a Ph.D. in his field, Dr. Lowemann has consistently been at the forefront of research and innovation, delving into ways to optimize human performance, cognition, and overall well-being. His work at the Institute revolves around a profound commitment to harnessing cutting-edge science and technology to help individuals lead more fulfilling and intelligent lives.
Dr. Lowemann’s influence extends to the educational platform BetterSmarter.me, where he shares his insights, findings, and personal development strategies with a broader audience. His ongoing mission is shaping the way we perceive and leverage the vast capacities of the human mind, offering invaluable contributions to society’s overall success and collective well-being.