Imagine you’re at a party where everyone is wearing masks, and you have to figure out who is who just by listening to their conversations and observing their actions. This is similar to the challenge that children face when learning new words. In the field of machine learning, researchers have developed multimodal neural networks that can learn to associate words with their meanings by analyzing both visual and linguistic information. These networks have shown promising results in tasks like image captioning and visual question answering. Now, scientists are exploring whether these neural networks can also explain how children learn words in real life. By comparing the behaviors of these networks to those observed in psychological studies on word learning, they found that the networks can indeed learn word-referent mappings from a limited amount of training, similar to what is seen in experimental settings. However, the networks were not able to capture all the phenomena associated with word learning, particularly those related to reasoning via mutual exclusivity. These findings shed light on the abilities and limitations of neural network algorithms in understanding word learning processes. To dive deeper into this fascinating research, check out the full article!
Abstract
In order to learn the mappings from words to referents, children must integrate co-occurrence information across individually ambiguous pairs of scenes and utterances, a challenge known as cross-situational word learning. In machine learning, recent multimodal neural networks have been shown to learn meaningful visual-linguistic mappings from cross-situational data, as needed to solve problems such as image captioning and visual question answering. These networks are potentially appealing as cognitive models because they can learn from raw visual and linguistic stimuli, something previous cognitive models have not addressed. In this paper, we examine whether recent machine learning approaches can help explain various behavioral phenomena from the psychological literature on cross-situational word learning. We consider two variants of a multimodal neural network architecture and look at seven different phenomena associated with cross-situational word learning and word learning more generally. Our results show that these networks can learn word-referent mappings from a single epoch of training, mimicking the amount of training commonly found in cross-situational word learning experiments. Additionally, these networks capture some, but not all of the phenomena we studied, with all of the failures related to reasoning via mutual exclusivity. These results provide insight into the kinds of phenomena that arise naturally from relatively generic neural network learning algorithms, and which word learning phenomena require additional inductive biases.
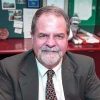
Dr. David Lowemann, M.Sc, Ph.D., is a co-founder of the Institute for the Future of Human Potential, where he leads the charge in pioneering Self-Enhancement Science for the Success of Society. With a keen interest in exploring the untapped potential of the human mind, Dr. Lowemann has dedicated his career to pushing the boundaries of human capabilities and understanding.
Armed with a Master of Science degree and a Ph.D. in his field, Dr. Lowemann has consistently been at the forefront of research and innovation, delving into ways to optimize human performance, cognition, and overall well-being. His work at the Institute revolves around a profound commitment to harnessing cutting-edge science and technology to help individuals lead more fulfilling and intelligent lives.
Dr. Lowemann’s influence extends to the educational platform BetterSmarter.me, where he shares his insights, findings, and personal development strategies with a broader audience. His ongoing mission is shaping the way we perceive and leverage the vast capacities of the human mind, offering invaluable contributions to society’s overall success and collective well-being.