Imagine you’re trying to solve a puzzle with two separate sets of clues. One set gives you information about how the pieces fit together, while the other set tells you about the colors and shapes of the pieces. Individually, they only give you part of the picture. But what if you could combine these clues to form a complete understanding? That’s exactly what scientists are doing in their quest to detect major depressive disorder (MDD) using neuroimaging data. In a groundbreaking study, researchers have developed an adaptive multimodal neuroimage integration (AMNI) framework that combines functional and structural MRI scans to improve MDD detection. By analyzing both the connectivity patterns in the brain and the physical structure of the brain, this innovative approach provides a more comprehensive understanding of MDD than ever before. The researchers found that by integrating these two modalities and considering their complementary information, they were able to achieve more accurate and reliable MDD detection. This breakthrough has the potential to revolutionize the diagnosis and treatment of MDD, leading to improved outcomes for individuals suffering from this debilitating condition. If you’re curious to dive deeper into this fascinating research, check out the full article!
Major depressive disorder (MDD) is one of the most common mental health disorders that can affect sleep, mood, appetite, and behavior of people. Multimodal neuroimaging data, such as functional and structural magnetic resonance imaging (MRI) scans, have been widely used in computer-aided detection of MDD. However, previous studies usually treat these two modalities separately, without considering their potentially complementary information. Even though a few studies propose integrating these two modalities, they usually suffer from significant inter-modality data heterogeneity. In this paper, we propose an adaptive multimodal neuroimage integration (AMNI) framework for automated MDD detection based on functional and structural MRIs. The AMNI framework consists of four major components: (1) a graph convolutional network to learn feature representations of functional connectivity networks derived from functional MRIs, (2) a convolutional neural network to learn features of T1-weighted structural MRIs, (3) a feature adaptation module to alleviate inter-modality difference, and (4) a feature fusion module to integrate feature representations extracted from two modalities for classification. To the best of our knowledge, this is among the first attempts to adaptively integrate functional and structural MRIs for neuroimaging-based MDD analysis by explicitly alleviating inter-modality heterogeneity. Extensive evaluations are performed on 533 subjects with resting-state functional MRI and T1-weighted MRI, with results suggesting the efficacy of the proposed method.
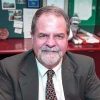
Dr. David Lowemann, M.Sc, Ph.D., is a co-founder of the Institute for the Future of Human Potential, where he leads the charge in pioneering Self-Enhancement Science for the Success of Society. With a keen interest in exploring the untapped potential of the human mind, Dr. Lowemann has dedicated his career to pushing the boundaries of human capabilities and understanding.
Armed with a Master of Science degree and a Ph.D. in his field, Dr. Lowemann has consistently been at the forefront of research and innovation, delving into ways to optimize human performance, cognition, and overall well-being. His work at the Institute revolves around a profound commitment to harnessing cutting-edge science and technology to help individuals lead more fulfilling and intelligent lives.
Dr. Lowemann’s influence extends to the educational platform BetterSmarter.me, where he shares his insights, findings, and personal development strategies with a broader audience. His ongoing mission is shaping the way we perceive and leverage the vast capacities of the human mind, offering invaluable contributions to society’s overall success and collective well-being.