Imagine you have a superpower that allows you to see inside the minds of people and diagnose their mental health conditions. Well, that’s essentially what machine-learning techniques combined with Electroencephalography (EEG) can do! A group of scientists wanted to improve the accuracy of diagnosing post-traumatic stress disorder (PTSD) using EEG data. They discovered that certain low-frequency brain waves, like the gentle ripples on a calm lake, held the key to better diagnosis. By analyzing six different frequency bands, they found that slower frequencies, particularly delta and theta waves, were the most effective in distinguishing between PTSD patients and healthy individuals. In fact, using the power spectrum density (PSD) features extracted from these slow waves resulted in an impressive 86.61% accuracy rate, surpassing previous studies by a whopping 13%. These findings provide important insights into the neurophysiology of PTSD and offer hope for developing a reliable computer-aided diagnosis (CAD) system that can assist clinicians in accurately identifying this condition. So if you’re curious about how science can decode brain waves and help detect mental health disorders, dive into the fascinating research behind EEG-based diagnosis of PTSD!
Electroencephalography (EEG)-based diagnosis of psychiatric diseases using machine-learning approaches has made possible the objective diagnosis of various psychiatric diseases. The objective of this study was to improve the performance of a resting-state EEG-based computer-aided diagnosis (CAD) system to diagnose post-traumatic stress disorder (PTSD), by optimizing the frequency bands used to extract EEG features. We used eyes-closed resting-state EEG data recorded from 77 PTSD patients and 58 healthy controls (HC). Source-level power spectrum densities (PSDs) of the resting-state EEG data were extracted from 6 frequency bands (delta, theta, alpha, low-beta, high-beta, and gamma), and the PSD features of each frequency band and their combinations were independently used to discriminate PTSD and HC. The classification performance was evaluated using support vector machine with leave-one-out cross validation. The PSD features extracted from slower-frequency bands (delta and theta) showed significantly higher classification performance than those of relatively higher-frequency bands. The best classification performance was achieved when using delta PSD features (86.61%), which was significantly higher than that reported in a recent study by about 13%. The PSD features selected to obtain better classification performances could be explained from a neurophysiological point of view, demonstrating the promising potential to develop a clinically reliable EEG-based CAD system for PTSD diagnosis.
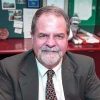
Dr. David Lowemann, M.Sc, Ph.D., is a co-founder of the Institute for the Future of Human Potential, where he leads the charge in pioneering Self-Enhancement Science for the Success of Society. With a keen interest in exploring the untapped potential of the human mind, Dr. Lowemann has dedicated his career to pushing the boundaries of human capabilities and understanding.
Armed with a Master of Science degree and a Ph.D. in his field, Dr. Lowemann has consistently been at the forefront of research and innovation, delving into ways to optimize human performance, cognition, and overall well-being. His work at the Institute revolves around a profound commitment to harnessing cutting-edge science and technology to help individuals lead more fulfilling and intelligent lives.
Dr. Lowemann’s influence extends to the educational platform BetterSmarter.me, where he shares his insights, findings, and personal development strategies with a broader audience. His ongoing mission is shaping the way we perceive and leverage the vast capacities of the human mind, offering invaluable contributions to society’s overall success and collective well-being.