Imagine you’re a detective, trying to solve a complex case. You have to piece together clues from different sources to unravel the mystery. That’s exactly what scientists do when studying brain oscillations. These oscillations, like rhythmic waves in the brain, play a crucial role in neural activity. To understand and analyze these oscillations, scientists use time-frequency representations (TFRs), which help them visualize how different frequencies change over time. But with so many methods available, how do they know which one is better? It’s like choosing the right tool from a toolbox. In this study, researchers introduce a methodology that evaluates the ‘quality’ of TFRs by quantifying how much information they retain about experimental conditions. By using advanced techniques like the superlet transform, they found that some methods provide more informative TFRs for complex brain signals. They also developed a method to measure the contribution of different time-frequency components to accurate discrimination among experimental conditions. This research opens doors to better understand brain dynamics and may lead to new discoveries in neural data analysis. If you’re curious about the fascinating world of brain oscillations and want to delve deeper into this groundbreaking research, check out the link below!
Brain oscillations are thought to subserve important functions by organizing the dynamical landscape of neural circuits. The expression of such oscillations in neural signals is usually evaluated using time-frequency representations (TFR), which resolve oscillatory processes in both time and frequency. While a vast number of methods exist to compute TFRs, there is often no objective criterion to decide which one is better. In feature-rich data, such as that recorded from the brain, sources of noise and unrelated processes abound and contaminate results. The impact of these distractor sources is especially problematic, such that TFRs that are more robust to contaminants are expected to provide more useful representations. In addition, the minutiae of the techniques themselves impart better or worse time and frequency resolutions, which also influence the usefulness of the TFRs. Here, we introduce a methodology to evaluate the “quality” of TFRs of neural signals by quantifying how much information they retain about the experimental condition during visual stimulation and recognition tasks, in mice and humans, respectively. We used machine learning to discriminate between various experimental conditions based on TFRs computed with different methods. We found that various methods provide more or less informative TFRs depending on the characteristics of the data. In general, however, more advanced techniques, such as the superlet transform, seem to provide better results for complex time-frequency landscapes, such as those extracted from electroencephalography signals. Finally, we introduce a method based on feature perturbation that is able to quantify how much time-frequency components contribute to the correct discrimination among experimental conditions. The methodology introduced in the present study may be extended to other analyses of neural data, enabling the discovery of data features that are modulated by the experimental manipulation.
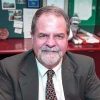
Dr. David Lowemann, M.Sc, Ph.D., is a co-founder of the Institute for the Future of Human Potential, where he leads the charge in pioneering Self-Enhancement Science for the Success of Society. With a keen interest in exploring the untapped potential of the human mind, Dr. Lowemann has dedicated his career to pushing the boundaries of human capabilities and understanding.
Armed with a Master of Science degree and a Ph.D. in his field, Dr. Lowemann has consistently been at the forefront of research and innovation, delving into ways to optimize human performance, cognition, and overall well-being. His work at the Institute revolves around a profound commitment to harnessing cutting-edge science and technology to help individuals lead more fulfilling and intelligent lives.
Dr. Lowemann’s influence extends to the educational platform BetterSmarter.me, where he shares his insights, findings, and personal development strategies with a broader audience. His ongoing mission is shaping the way we perceive and leverage the vast capacities of the human mind, offering invaluable contributions to society’s overall success and collective well-being.