Imagine you’re at a buffet, but each dish is cooked by a different chef. The flavors and styles may vary, creating what we call ‘batch effects.’ In the exciting field of deep learning applied to biomedical research, these batch effects can create challenges when training models with neuroimaging data. But fear not! Researchers have developed multi-source domain adaptation (MSDA) techniques to overcome this hurdle. They combine fMRI data from multiple sites to create a large and diverse dataset, but how do they ensure accurate model predictions? This comparative study examines popular MSDA methods like MDAN, DARN, MDMN, and M3SDA. The study analyzes their performance in predicting different labels in neuroimaging data, such as illness, age, and sex. It also considers factors like class imbalance and the number of sites. The results highlight the effectiveness of MSDA models in improving classification accuracy and their potential impact on advancing neuroimaging-based applications. So grab your lab coat and dive into the full article to discover how MSDA techniques are powering up neuroimaging research!
The past decade has seen an increasing number of applications of deep learning (DL) techniques to biomedical fields, especially in neuroimaging-based analysis. Such DL-based methods are generally data-intensive and require a large number of training instances, which might be infeasible to acquire from a single acquisition site, especially for data, such as fMRI scans, due to the time and costs that they demand. We can attempt to address this issue by combining fMRI data from various sites, thereby creating a bigger heterogeneous dataset. Unfortunately, the inherent differences in the combined data, known as batch effects, often hamper learning a model. To mitigate this issue, techniques such as multi-source domain adaptation [Multi-source Domain Adversarial Networks (MSDA)] aim at learning an effective classification function that uses (learned) domain-invariant latent features. This article analyzes and compares the performance of various popular MSDA methods [MDAN, Domain AggRegation Networks (DARN), Multi-Domain Matching Networks (MDMN), and Moment Matching for MSDA (M3SDA)] at predicting different labels (illness, age, and sex) of images from two public rs-fMRI datasets: ABIDE 1and ADHD-200. It also evaluates the impact of various conditions such as class imbalance, the number of sites along with a comparison of the degree of adaptation of each of the methods, thereby presenting the effectiveness of MSDA models in neuroimaging-based applications.
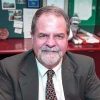
Dr. David Lowemann, M.Sc, Ph.D., is a co-founder of the Institute for the Future of Human Potential, where he leads the charge in pioneering Self-Enhancement Science for the Success of Society. With a keen interest in exploring the untapped potential of the human mind, Dr. Lowemann has dedicated his career to pushing the boundaries of human capabilities and understanding.
Armed with a Master of Science degree and a Ph.D. in his field, Dr. Lowemann has consistently been at the forefront of research and innovation, delving into ways to optimize human performance, cognition, and overall well-being. His work at the Institute revolves around a profound commitment to harnessing cutting-edge science and technology to help individuals lead more fulfilling and intelligent lives.
Dr. Lowemann’s influence extends to the educational platform BetterSmarter.me, where he shares his insights, findings, and personal development strategies with a broader audience. His ongoing mission is shaping the way we perceive and leverage the vast capacities of the human mind, offering invaluable contributions to society’s overall success and collective well-being.