Just like a symphony, the firing patterns of neuronal populations can come together in a beautifully synchronized dance. Scientists are delving into how these collective oscillations emerge and how adding a touch of randomness can actually enhance their regularity. In this study, researchers focused on coherence resonance, which refers to the phenomenon where introducing noise leads to more organized spiking activity in neurons. Using a two-layered network model with excitatory and inhibitory neurons, they explored how different factors influence coherence resonance. By tweaking the proportion of inhibitory neurons, the strength of interlayer connections, and the architecture of interactions between different neuron types, they uncovered key determinants that impact regularity. For instance, increasing the number of inhibitory neurons or enhancing their connections can boost the synchrony of neural activity. Furthermore, they found that a stronger damping effect in the excitatory layer also contributes to greater regularity. These findings shed light on how neural networks process information and could inspire the development of more efficient artificial neural circuits by leveraging noise-induced effects. To dive deeper into this exciting research, check out the full article!
The firing patterns of neuronal populations often exhibit emergent collective oscillations, which can display substantial regularity even though the dynamics of individual elements is very stochastic. One of the many phenomena that is often studied in this context is coherence resonance, where additional noise leads to improved regularity of spiking activity in neurons. In this work, we investigate how the coherence resonance phenomenon manifests itself in populations of excitatory and inhibitory neurons. In our simulations, we use the coupled FitzHugh-Nagumo oscillators in the excitable regime and in the presence of neuronal noise. Formally, our model is based on the concept of a two-layered network, where one layer contains inhibitory neurons, the other excitatory neurons, and the interlayer connections represent heterotypic interactions. The neuronal activity is simulated in realistic coupling schemes in which neurons within each layer are connected with undirected connections, whereas neurons of different types are connected with directed interlayer connections. In this setting, we investigate how different neurophysiological determinants affect the coherence resonance. Specifically, we focus on the proportion of inhibitory neurons, the proportion of excitatory interlayer axons, and the architecture of interlayer connections between inhibitory and excitatory neurons. Our results reveal that the regularity of simulated neural activity can be increased by a stronger damping of the excitatory layer. This can be accomplished with a higher proportion of inhibitory neurons, a higher fraction of inhibitory interlayer axons, a stronger coupling between inhibitory axons, or by a heterogeneous configuration of interlayer connections. Our approach of modeling multilayered neuronal networks in combination with stochastic dynamics offers a novel perspective on how the neural architecture can affect neural information processing and provide possible applications in designing networks of artificial neural circuits to optimize their function via noise-induced phenomena.
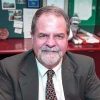
Dr. David Lowemann, M.Sc, Ph.D., is a co-founder of the Institute for the Future of Human Potential, where he leads the charge in pioneering Self-Enhancement Science for the Success of Society. With a keen interest in exploring the untapped potential of the human mind, Dr. Lowemann has dedicated his career to pushing the boundaries of human capabilities and understanding.
Armed with a Master of Science degree and a Ph.D. in his field, Dr. Lowemann has consistently been at the forefront of research and innovation, delving into ways to optimize human performance, cognition, and overall well-being. His work at the Institute revolves around a profound commitment to harnessing cutting-edge science and technology to help individuals lead more fulfilling and intelligent lives.
Dr. Lowemann’s influence extends to the educational platform BetterSmarter.me, where he shares his insights, findings, and personal development strategies with a broader audience. His ongoing mission is shaping the way we perceive and leverage the vast capacities of the human mind, offering invaluable contributions to society’s overall success and collective well-being.