Alzheimer’s disease is like a complex maze that poses a significant challenge for healthcare professionals and researchers. With its diverse symptoms and limited accuracy of neuroimaging techniques, early and accurate diagnosis is crucial. In this article, the researchers built a brain network using various neuroimaging data such as MRI, DWI, and PET scans to understand the distinct burden of AD on different brain regions, the lateralization of brain networks between left and right hemispheres, and the asymmetry of AD attacks. To tackle this intricate problem, they developed a regional brain fusion-graph convolutional network (RBF-GCN) that incorporates a framework called RBF along with three essential modules. One of these modules involves an adaptive native node attribute (ANNA) unit which enhances the performance of the network effectively. Through numerous experiments using the ADNI clinical dataset, the researchers not only validated the effectiveness of their approach but also achieved competitive results in classifying AD stages. This groundbreaking research provides valuable insights into improving Alzheimer’s diagnosis and opens up new opportunities for preventing and treating this debilitating disease.
Alzheimer’s disease (AD) has raised extensive concern in healthcare and academia as one of the most prevalent health threats to the elderly. Due to the irreversible nature of AD, early and accurate diagnoses are significant for effective prevention and treatment. However, diverse clinical symptoms and limited neuroimaging accuracy make diagnoses challenging. In this article, we built a brain network for each subject, which assembles several commonly used neuroimaging data simply and reasonably, including structural magnetic resonance imaging (MRI), diffusion-weighted imaging (DWI), and amyloid positron emission tomography (PET). Based on some existing research results, we applied statistical methods to analyze (i) the distinct affinity of AD burden on each brain region, (ii) the topological lateralization between left and right hemispheric sub-networks, and (iii) the asymmetry of the AD attacks on the left and right hemispheres. In the light of advances in graph convolutional networks for graph classifications and summarized characteristics of brain networks and AD pathologies, we proposed a regional brain fusion-graph convolutional network (RBF-GCN), which is constructed with an RBF framework mainly, including three sub-modules, namely, hemispheric network generation module, multichannel GCN module, and feature fusion module. In the multichannel GCN module, the improved GCN by our proposed adaptive native node attribute (ANNA) unit embeds within each channel independently. We not only fully verified the effectiveness of the RBF framework and ANNA unit but also achieved competitive results in multiple sets of AD stages’ classification tasks using hundreds of experiments over the ADNI clinical dataset.
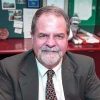
Dr. David Lowemann, M.Sc, Ph.D., is a co-founder of the Institute for the Future of Human Potential, where he leads the charge in pioneering Self-Enhancement Science for the Success of Society. With a keen interest in exploring the untapped potential of the human mind, Dr. Lowemann has dedicated his career to pushing the boundaries of human capabilities and understanding.
Armed with a Master of Science degree and a Ph.D. in his field, Dr. Lowemann has consistently been at the forefront of research and innovation, delving into ways to optimize human performance, cognition, and overall well-being. His work at the Institute revolves around a profound commitment to harnessing cutting-edge science and technology to help individuals lead more fulfilling and intelligent lives.
Dr. Lowemann’s influence extends to the educational platform BetterSmarter.me, where he shares his insights, findings, and personal development strategies with a broader audience. His ongoing mission is shaping the way we perceive and leverage the vast capacities of the human mind, offering invaluable contributions to society’s overall success and collective well-being.