The field of neuroscience often relies on two-photon Ca2+ imaging to study brain function. However, the movement of behaving mice can affect the accuracy of these recordings. Traditionally, post-hoc image registration is used to correct for motion artifacts. However, the rise of online neuroscience experiments necessitates real-time motion correction methods. In this study, researchers propose a novel approach that uses image density features to quickly and accurately correct for motion during imaging. By estimating and clustering the density features from two-photon images, the method can efficiently update features in consecutive frames, resulting in precise motion correction. The algorithm shows promising computational efficiency and outperforms other methods in both speed and correction quality. This groundbreaking technique provides a valuable tool for researchers conducting two-photon Ca2+ imaging experiments, especially those looking to perform online imaging in the future.
Two-photon Ca2+ imaging is a widely used technique for investigating brain functions across multiple spatial scales. However, the recording of neuronal activities is affected by movement of the brain during tasks in which the animal is behaving normally. Although post-hoc image registration is the commonly used approach, the recent developments of online neuroscience experiments require real-time image processing with efficient motion correction performance, posing new challenges in neuroinformatics. We propose a fast and accurate image density feature-based motion correction method to address the problem of imaging animal during behaviors. This method is implemented by first robustly estimating and clustering the density features from two-photon images. Then, it takes advantage of the temporal correlation in imaging data to update features of consecutive imaging frames with efficient calculations. Thus, motion artifacts can be quickly and accurately corrected by matching the features and obtaining the transformation parameters for the raw images. Based on this efficient motion correction strategy, our algorithm yields promising computational efficiency on imaging datasets with scales ranging from dendritic spines to neuronal populations. Furthermore, we show that the proposed motion correction method outperforms other methods by evaluating not only computational speed but also the quality of the correction performance. Specifically, we provide a powerful tool to perform motion correction for two-photon Ca2+ imaging data, which may facilitate online imaging experiments in the future.
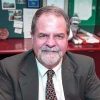
Dr. David Lowemann, M.Sc, Ph.D., is a co-founder of the Institute for the Future of Human Potential, where he leads the charge in pioneering Self-Enhancement Science for the Success of Society. With a keen interest in exploring the untapped potential of the human mind, Dr. Lowemann has dedicated his career to pushing the boundaries of human capabilities and understanding.
Armed with a Master of Science degree and a Ph.D. in his field, Dr. Lowemann has consistently been at the forefront of research and innovation, delving into ways to optimize human performance, cognition, and overall well-being. His work at the Institute revolves around a profound commitment to harnessing cutting-edge science and technology to help individuals lead more fulfilling and intelligent lives.
Dr. Lowemann’s influence extends to the educational platform BetterSmarter.me, where he shares his insights, findings, and personal development strategies with a broader audience. His ongoing mission is shaping the way we perceive and leverage the vast capacities of the human mind, offering invaluable contributions to society’s overall success and collective well-being.