The brain is a complex network, like a bustling city with countless streets and intersections. Scientists have made great progress in mapping out the brain’s neural architecture, but understanding how its microscale physiology affects its macroscale dynamics remains a challenge. To bridge this gap, researchers have developed a mathematical model called a neural-mass model to study brain dynamics. In this study, scientists created a neural-mass model of the mouse cortex and used bifurcation diagrams to analyze its dynamics. Bifurcation diagrams act like a roadmap, showing how different brain regions respond to inputs and vary in their dynamics. Surprisingly, the researchers discovered that even simple dynamical regimes could generate strong fits to resting-state functional magnetic resonance imaging (fMRI) data. These findings suggest that the structure of direct connections between brain regions plays a crucial role in shaping brain activity. Furthermore, by perturbing the coupling strengths across the cortex based on cell-density data, the researchers observed spatially dependent constraints on cortical activity and a greater variety of dynamical regimes. This study demonstrates an important approach for understanding brain dynamics and paves the way for building models that explain the fundamental principles underlying large-scale brain activity.
New brain atlases with high spatial resolution and whole-brain coverage have rapidly advanced our knowledge of the brain’s neural architecture, including the systematic variation of excitatory and inhibitory cell densities across the mammalian cortex. But understanding how the brain’s microscale physiology shapes brain dynamics at the macroscale has remained a challenge. While physiologically based mathematical models of brain dynamics are well placed to bridge this explanatory gap, their complexity can form a barrier to providing clear mechanistic interpretation of the dynamics they generate. In this work, we develop a neural-mass model of the mouse cortex and show how bifurcation diagrams, which capture local dynamical responses to inputs and their variation across brain regions, can be used to understand the resulting whole-brain dynamics. We show that strong fits to resting-state functional magnetic resonance imaging (fMRI) data can be found in surprisingly simple dynamical regimes—including where all brain regions are confined to a stable fixed point—in which regions are able to respond strongly to variations in their inputs, consistent with direct structural connections providing a strong constraint on functional connectivity in the anesthetized mouse. We also use bifurcation diagrams to show how perturbations to local excitatory and inhibitory coupling strengths across the cortex, constrained by cell-density data, provide spatially dependent constraints on resulting cortical activity, and support a greater diversity of coincident dynamical regimes. Our work illustrates methods for visualizing and interpreting model performance in terms of underlying dynamical mechanisms, an approach that is crucial for building explanatory and physiologically grounded models of the dynamical principles that underpin large-scale brain activity.
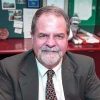
Dr. David Lowemann, M.Sc, Ph.D., is a co-founder of the Institute for the Future of Human Potential, where he leads the charge in pioneering Self-Enhancement Science for the Success of Society. With a keen interest in exploring the untapped potential of the human mind, Dr. Lowemann has dedicated his career to pushing the boundaries of human capabilities and understanding.
Armed with a Master of Science degree and a Ph.D. in his field, Dr. Lowemann has consistently been at the forefront of research and innovation, delving into ways to optimize human performance, cognition, and overall well-being. His work at the Institute revolves around a profound commitment to harnessing cutting-edge science and technology to help individuals lead more fulfilling and intelligent lives.
Dr. Lowemann’s influence extends to the educational platform BetterSmarter.me, where he shares his insights, findings, and personal development strategies with a broader audience. His ongoing mission is shaping the way we perceive and leverage the vast capacities of the human mind, offering invaluable contributions to society’s overall success and collective well-being.