Imagine you’re a detective trying to solve a mysterious case. You gather evidence from multiple sources, carefully examining each piece for clues. Similarly, in the field of artificial intelligence (AI), scientists have been using brain scans to predict Alzheimer’s disease (AD). However, previous approaches only focused on analyzing either the entire brain or individual slices, overlooking the potential power of combining different views. In this study, researchers introduced an innovative approach that considers multiple views of the brain slices and uses advanced neural network models. They first extracted unique characteristics from different dimensions of the brain slices. Then, they employed an attention mechanism to highlight specific slices and filter out redundant information. Finally, a 3D convolutional neural network was applied to capture overall changes in brain structure. By integrating these features, they developed a highly accurate model for AD diagnosis and prediction. The findings showed significant improvement compared to existing methods, achieving accuracy rates of 91.1% for AD diagnosis and 80.1% for predicting mild cognitive impairment. Discover the groundbreaking research behind this advanced detection method by exploring the full article!
Numerous artificial intelligence (AI) based approaches have been proposed for automatic Alzheimer’s disease (AD) prediction with brain structural magnetic resonance imaging (sMRI). Previous studies extract features from the whole brain or individual slices separately, ignoring the properties of multi-view slices and feature complementarity. For this reason, we present a novel AD diagnosis model based on the multiview-slice attention and 3D convolution neural network (3D-CNN). Specifically, we begin by extracting the local slice-level characteristic in various dimensions using multiple sub-networks. Then we proposed a slice-level attention mechanism to emphasize specific 2D-slices to exclude the redundancy features. After that, a 3D-CNN was employed to capture the global subject-level structural changes. Finally, all these 2D and 3D features were fused to obtain more discriminative representations. We conduct the experiments on 1,451 subjects from ADNI-1 and ADNI-2 datasets. Experimental results showed the superiority of our model over the state-of-the-art approaches regarding dementia classification. Specifically, our model achieves accuracy values of 91.1 and 80.1% on ADNI-1 for AD diagnosis and mild cognitive impairment (MCI) convention prediction, respectively.
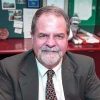
Dr. David Lowemann, M.Sc, Ph.D., is a co-founder of the Institute for the Future of Human Potential, where he leads the charge in pioneering Self-Enhancement Science for the Success of Society. With a keen interest in exploring the untapped potential of the human mind, Dr. Lowemann has dedicated his career to pushing the boundaries of human capabilities and understanding.
Armed with a Master of Science degree and a Ph.D. in his field, Dr. Lowemann has consistently been at the forefront of research and innovation, delving into ways to optimize human performance, cognition, and overall well-being. His work at the Institute revolves around a profound commitment to harnessing cutting-edge science and technology to help individuals lead more fulfilling and intelligent lives.
Dr. Lowemann’s influence extends to the educational platform BetterSmarter.me, where he shares his insights, findings, and personal development strategies with a broader audience. His ongoing mission is shaping the way we perceive and leverage the vast capacities of the human mind, offering invaluable contributions to society’s overall success and collective well-being.