Imagine you’re on a treasure hunt, but instead of searching for gold, you’re searching for abnormal brain activity using functional magnetic resonance imaging (fMRI). It’s like having a map that shows you exactly where the buried treasure is hidden in your brain! But here’s the catch: most studies focus on large areas of the brain, while few have explored the tiny details of single voxels. That’s where our team comes in. We developed a clever 1D convolutional neural network (1D-CNN) that analyzes the temporal dynamics of single-voxel fMRI time-series data. By using wavelet transformation, we transformed these time-series into a frequency domain, allowing us to identify different task states with incredible accuracy. In fact, our 1D-CNN model outperformed traditional analyses by decoding task states with an impressive 94% accuracy! And not only that, but our approach also paves the way for pinpointing abnormal brain activity and potentially guiding precision brain stimulation therapy. So grab your shovels and dive into the exciting world of single-voxel analysis with 1D-CNN and wavelet transformation!
Functional magnetic resonance imaging (fMRI) is one of the best techniques for precise localization of abnormal brain activity non-invasively. Machine-learning approaches have been widely used in neuroimaging studies; however, few studies have investigated the single-voxel modeling of fMRI data under cognitive tasks. We proposed a hybrid one-dimensional (1D) convolutional neural network (1D-CNN) based on the temporal dynamics of single-voxel fMRI time-series and successfully differentiated two continuous task states, namely, self-initiated (SI) and visually guided (VG) motor tasks. First, 25 activation peaks were identified from the contrast maps of SI and VG tasks in a blocked design. Then, the fMRI time-series of each peak voxel was transformed into a temporal-frequency domain by using continuous wavelet transform across a broader frequency range (0.003–0.313 Hz, with a step of 0.01 Hz). The transformed time-series was inputted into a 1D-CNN model for the binary classification of SI and VG continuous tasks. Compared with the univariate analysis, e.g., amplitude of low-frequency fluctuation (ALFF) at each frequency band, including, wavelet-ALFF, the 1D-CNN model highly outperformed wavelet-ALFF, with more efficient decoding models [46% of 800 models showing area under the curve (AUC) > 0.61] and higher decoding accuracies (94% of the efficient models), especially on the high-frequency bands (>0.1 Hz). Moreover, our results also demonstrated the advantages of wavelet decompositions over the original fMRI series by showing higher decoding performance on all peak voxels. Overall, this study suggests a great potential of single-voxel analysis using 1D-CNN and wavelet transformation of fMRI series with continuous, naturalistic, steady-state task design or resting-state design. It opens new avenues to precise localization of abnormal brain activity and fMRI-guided precision brain stimulation therapy.
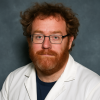
Dr. David Lowemann, M.Sc, Ph.D., is a co-founder of the Institute for the Future of Human Potential, where he leads the charge in pioneering Self-Enhancement Science for the Success of Society. With a keen interest in exploring the untapped potential of the human mind, Dr. Lowemann has dedicated his career to pushing the boundaries of human capabilities and understanding.
Armed with a Master of Science degree and a Ph.D. in his field, Dr. Lowemann has consistently been at the forefront of research and innovation, delving into ways to optimize human performance, cognition, and overall well-being. His work at the Institute revolves around a profound commitment to harnessing cutting-edge science and technology to help individuals lead more fulfilling and intelligent lives.
Dr. Lowemann’s influence extends to the educational platform BetterSmarter.me, where he shares his insights, findings, and personal development strategies with a broader audience. His ongoing mission is shaping the way we perceive and leverage the vast capacities of the human mind, offering invaluable contributions to society’s overall success and collective well-being.