Imagine a group of neurons constantly fine-tuning their parameters to perfectly capture and encode the ever-changing visuals around them. This is precisely what this study explores – a self-tuning mechanism that allows neurons to adapt rapidly to varying visual stimuli. By leveraging efficient sensory encoding principles, the researchers demonstrate how neural tuning curve parameters can be continually updated to optimize the representation of recently detected stimulus values. They applied this concept to a neural model that estimates self-motion direction based on optic flow, and found that dynamically tuning the speed-sensitive units led to more accurate and faster estimates of heading compared to static tuning. The implications of this research go beyond just modeling biological visual systems; it opens up possibilities for improving artificial intelligence algorithms that rely on optic flow processing as well. If you’re fascinated by the brain’s ability to constantly adapt and optimize its perception of the world, dive into the full article for a deeper understanding of this dynamic efficient sensory encoding approach!
This paper introduces a self-tuning mechanism for capturing rapid adaptation to changing visual stimuli by a population of neurons. Building upon the principles of efficient sensory encoding, we show how neural tuning curve parameters can be continually updated to optimally encode a time-varying distribution of recently detected stimulus values. We implemented this mechanism in a neural model that produces human-like estimates of self-motion direction (i.e., heading) based on optic flow. The parameters of speed-sensitive units were dynamically tuned in accordance with efficient sensory encoding such that the network remained sensitive as the distribution of optic flow speeds varied. In two simulation experiments, we found that model performance with dynamic tuning yielded more accurate, shorter latency heading estimates compared to the model with static tuning. We conclude that dynamic efficient sensory encoding offers a plausible approach for capturing adaptation to varying visual environments in biological visual systems and neural models alike.
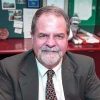
Dr. David Lowemann, M.Sc, Ph.D., is a co-founder of the Institute for the Future of Human Potential, where he leads the charge in pioneering Self-Enhancement Science for the Success of Society. With a keen interest in exploring the untapped potential of the human mind, Dr. Lowemann has dedicated his career to pushing the boundaries of human capabilities and understanding.
Armed with a Master of Science degree and a Ph.D. in his field, Dr. Lowemann has consistently been at the forefront of research and innovation, delving into ways to optimize human performance, cognition, and overall well-being. His work at the Institute revolves around a profound commitment to harnessing cutting-edge science and technology to help individuals lead more fulfilling and intelligent lives.
Dr. Lowemann’s influence extends to the educational platform BetterSmarter.me, where he shares his insights, findings, and personal development strategies with a broader audience. His ongoing mission is shaping the way we perceive and leverage the vast capacities of the human mind, offering invaluable contributions to society’s overall success and collective well-being.