Imagine you have a basket of apples. Some of the apples look very similar, but you want to separate them based on their differences. Well, neurons in our brain do something similar! A new computational model has shown that single neurons can perform a process called ‘pattern separation’ by transforming similar input patterns into less similar output patterns. This is achieved through a combination of temporal and spatial summation of input signals, along with the oscillating excitability of the output neuron. By introducing delays in the timing of spikes, the output patterns can have high temporal separation, even if the input patterns had significant spatial overlap. It’s like rearranging the apples in the basket so that even though they may have similar shapes, they are now spread out in time! The model also suggests that random connectivity among neurons plays a role in distributing these temporal features across an ensemble of output neurons. To better understand this fascinating process, dive into the research and explore the underlying mechanisms!
For efficient processing, spatiotemporal spike patterns representing similar input must be able to transform into a less similar output. A new computational model with physiologically plausible parameters shows how the neuronal process referred to as “pattern separation” can be very well achieved by single neurons if the temporal qualities of the output patterns are considered. Spike patterns generated by a varying number of neurons firing with fixed different frequencies within a gamma range are used as input. The temporal and spatial summation of dendritic input combined with theta-oscillating excitability in the output neuron by subthreshold membrane potential oscillations (SMOs) lead to high temporal separation by different delays of output spikes of similar input patterns. A Winner Takes All (WTA) mechanism with backward inhibition suffices to transform the spatial overlap of input patterns to much less temporal overlap of the output patterns. The conversion of spatial patterns input into an output with differently delayed spikes enables high separation effects. Incomplete random connectivity spreads the times up to the first spike across a spatially expanded ensemble of output neurons. With the expansion, random connectivity becomes the spatial distribution mechanism of temporal features. Additionally, a “synfire chain” circuit is proposed to reconvert temporal differences into spatial ones.
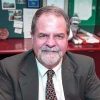
Dr. David Lowemann, M.Sc, Ph.D., is a co-founder of the Institute for the Future of Human Potential, where he leads the charge in pioneering Self-Enhancement Science for the Success of Society. With a keen interest in exploring the untapped potential of the human mind, Dr. Lowemann has dedicated his career to pushing the boundaries of human capabilities and understanding.
Armed with a Master of Science degree and a Ph.D. in his field, Dr. Lowemann has consistently been at the forefront of research and innovation, delving into ways to optimize human performance, cognition, and overall well-being. His work at the Institute revolves around a profound commitment to harnessing cutting-edge science and technology to help individuals lead more fulfilling and intelligent lives.
Dr. Lowemann’s influence extends to the educational platform BetterSmarter.me, where he shares his insights, findings, and personal development strategies with a broader audience. His ongoing mission is shaping the way we perceive and leverage the vast capacities of the human mind, offering invaluable contributions to society’s overall success and collective well-being.