Imagine a magical forest where each tree represents a different machine learning algorithm, and the trees work together to classify the causes of ischemic stroke. In this study, researchers trained and evaluated six ML models, including Random Forests, Logistic Regression, and Extreme Gradient Boosting. The goal? To accurately identify the etiology of stroke and improve prognosis and prevention strategies. Over 18,000 patients participated in the study, with data collected from two phases – one for algorithm development and one for testing. The Random Forests model emerged as the best performer, with impressive area under the curve (AUC) values for predicting cardioembolism, large-artery atherosclerosis, and small-artery occlusion. Key factors in distinguishing stroke causes were identified as atrial fibrillation and the degree of stenosis of intracranial arteries. This innovative RF model could become an essential tool for neurologists in categorizing stroke etiologies. To learn more about this cutting-edge research and its potential impact on stroke treatment and prevention, check out the full article!
BackgroundPrognosis, recurrence rate, and secondary prevention strategies differ by different etiologies in acute ischemic stroke. However, identifying its cause is challenging.ObjectiveThis study aimed to develop a model to identify the cause of stroke using machine learning (ML) methods and test its accuracy.MethodsWe retrospectively reviewed the data of patients who had determined etiology defined by the Trial of ORG 10172 in Acute Stroke Treatment (TOAST) from CASE-II (NCT04487340) to train and evaluate six ML models, namely, Random Forests (RF), Logistic Regression (LR), Extreme Gradient Boosting (XGBoost), K-Nearest Neighbor (KNN), Ada Boosting, Gradient Boosting Machine (GBM), for the detection of cardioembolism (CE), large-artery atherosclerosis (LAA), and small-artery occlusion (SAO). Between October 2016 and April 2020, patients were enrolled consecutively for algorithm development (phase one). Between June 2020 and December 2020, patients were enrolled consecutively in a test set for algorithm test (phase two). Area under the curve (AUC), precision, recall, accuracy, and F1 score were calculated for the prediction model.ResultsFinally, a total of 18,209 patients were enrolled in phase one, including 13,590 patients (i.e., 6,089 CE, 4,539 LAA, and 2,962 SAO) in the model, and a total of 3,688 patients were enrolled in phase two, including 3,070 patients (i.e., 1,103 CE, 1,269 LAA, and 698 SAO) in the model. Among the six models, the best models were RF, XGBoost, and GBM, and we chose the RF model as our final model. Based on the test set, the AUC values of the RF model to predict CE, LAA, and SAO were 0.981 (95%CI, 0.978–0.986), 0.919 (95%CI, 0.911–0.928), and 0.918 (95%CI, 0.908–0.927), respectively. The most important items to identify CE, LAA, and SAO were atrial fibrillation and degree of stenosis of intracranial arteries.ConclusionThe proposed RF model could be a useful diagnostic tool to help neurologists categorize etiologies of stroke.Clinical Trial Registration[www.ClinicalTrials.gov], identifier [NCT01274117].
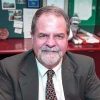
Dr. David Lowemann, M.Sc, Ph.D., is a co-founder of the Institute for the Future of Human Potential, where he leads the charge in pioneering Self-Enhancement Science for the Success of Society. With a keen interest in exploring the untapped potential of the human mind, Dr. Lowemann has dedicated his career to pushing the boundaries of human capabilities and understanding.
Armed with a Master of Science degree and a Ph.D. in his field, Dr. Lowemann has consistently been at the forefront of research and innovation, delving into ways to optimize human performance, cognition, and overall well-being. His work at the Institute revolves around a profound commitment to harnessing cutting-edge science and technology to help individuals lead more fulfilling and intelligent lives.
Dr. Lowemann’s influence extends to the educational platform BetterSmarter.me, where he shares his insights, findings, and personal development strategies with a broader audience. His ongoing mission is shaping the way we perceive and leverage the vast capacities of the human mind, offering invaluable contributions to society’s overall success and collective well-being.