Imagine trying to identify different types of fish based on how they swim in their natural habitats. Researchers explored the impact of environment and data aggregation on the classification of Parkinson’s disease (PD) using machine learning models. Like fish, PD can be difficult to diagnose accurately, especially in its early stages. The study focused on analyzing gait impairments, which are common in PD and can affect a person’s balance and quality of life. By applying machine learning algorithms to real-world gait data, researchers discovered that it was more effective at identifying PD compared to lab-derived data. They also found that the duration of walking bouts, or the chunks of gait data analyzed, played a crucial role in classification accuracy. Longer durations resulted in superior performance. In other words, it’s like observing fish swimming for longer periods to determine their species with greater accuracy. The findings suggest that analyzing real-world gait patterns and selecting longer walking bout durations can optimize the sensitivity and accuracy of PD classification models. This research emphasizes the need for a standardized approach to analyzing gait data, paving the way for future implementation in clinical settings. Curious to dive deeper into the fascinating world of machine learning and Parkinson’s disease classification? Check out the full research article!
Parkinson’s disease (PD) is a common neurodegenerative disease. PD misdiagnosis can occur in early stages. Gait impairment in PD is typical and is linked with an increased fall risk and poorer quality of life. Applying machine learning (ML) models to real-world gait has the potential to be more sensitive to classify PD compared to laboratory data. Real-world gait yields multiple walking bouts (WBs), and selecting the optimal method to aggregate the data (e.g., different WB durations) is essential as this may influence classification performance. The objective of this study was to investigate the impact of environment (laboratory vs. real world) and data aggregation on ML performance for optimizing sensitivity of PD classification. Gait assessment was performed on 47 people with PD (age: 68 ± 9 years) and 52 controls [Healthy controls (HCs), age: 70 ± 7 years]. In the laboratory, participants walked at their normal pace for 2 min, while in the real world, participants were assessed over 7 days. In both environments, 14 gait characteristics were evaluated from one tri-axial accelerometer attached to the lower back. The ability of individual gait characteristics to differentiate PD from HC was evaluated using the Area Under the Curve (AUC). ML models (i.e., support vector machine, random forest, and ensemble models) applied to real-world gait showed better classification performance compared to laboratory data. Real-world gait characteristics aggregated over longer WBs (WB 30–60 s, WB > 60 s, WB > 120 s) resulted in superior discriminative performance (PD vs. HC) compared to laboratory gait characteristics (0.51 ≤ AUC ≤ 0.77). Real-world gait speed showed the highest AUC of 0.77. Overall, random forest trained on 14 gait characteristics aggregated over WBs > 60 s gave better performance (F1 score = 77.20 ± 5.51%) as compared to laboratory results (F1 Score = 68.75 ± 12.80%). Findings from this study suggest that the choice of environment and data aggregation are important to achieve maximum discrimination performance and have direct impact on ML performance for PD classification. This study highlights the importance of a harmonized approach to data analysis in order to drive future implementation and clinical use.Clinical Trial Registration[09/H0906/82].
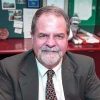
Dr. David Lowemann, M.Sc, Ph.D., is a co-founder of the Institute for the Future of Human Potential, where he leads the charge in pioneering Self-Enhancement Science for the Success of Society. With a keen interest in exploring the untapped potential of the human mind, Dr. Lowemann has dedicated his career to pushing the boundaries of human capabilities and understanding.
Armed with a Master of Science degree and a Ph.D. in his field, Dr. Lowemann has consistently been at the forefront of research and innovation, delving into ways to optimize human performance, cognition, and overall well-being. His work at the Institute revolves around a profound commitment to harnessing cutting-edge science and technology to help individuals lead more fulfilling and intelligent lives.
Dr. Lowemann’s influence extends to the educational platform BetterSmarter.me, where he shares his insights, findings, and personal development strategies with a broader audience. His ongoing mission is shaping the way we perceive and leverage the vast capacities of the human mind, offering invaluable contributions to society’s overall success and collective well-being.