Imagine you’re playing a video game and trying to guide your character through a complex maze. The challenge lies in controlling the cursor on the screen accurately and smoothly using only your brain signals. This article introduces an innovative breakthrough in brain-computer interface technology called the spectral-temporal long short-term memory (stLSTM) network. It’s like having a high-tech controller for your brain! By analyzing the different frequencies of brain waves, the stLSTM network can decode your intentions and detect any errors in your cursor’s movement. It combines these spectral and temporal features to generate precise control signals for both the vertical and horizontal directions of the cursor, resulting in improved accuracy. In fact, compared to existing methods, this new framework reduces the root mean square error (RMSE) by an average of 63.45%! The visual representation of the cursor’s actual trajectory also shows that this technology allows for more accurate control in complex tasks. If you’re fascinated by neuroscience and want to learn more about this cutting-edge research, check out the full article.
Two-dimensional cursor control is an important and challenging problem in the field of electroencephalography (EEG)-based brain computer interfaces (BCIs) applications. However, most BCIs based on categorical outputs are incapable of generating accurate and smooth control trajectories. In this article, a novel EEG decoding framework based on a spectral-temporal long short-term memory (stLSTM) network is proposed to generate control signals in the horizontal and vertical directions for accurate cursor control. Precisely, the spectral information is used to decode the subject’s motor imagery intention, and the error-related P300 information is used to detect a deviation in the movement trajectory. The concatenated spectral and temporal features are fed into the stLSTM network and mapped to the velocities in vertical and horizontal directions of the 2D cursor under the velocity-constrained (VC) strategy, which enables the decoding network to fit the velocity in the imaginary direction and simultaneously suppress the velocity in the non-imaginary direction. This proposed framework was validated on a public real BCI control dataset. Results show that compared with the state-of-the-art method, the RMSE of the proposed method in the non-imaginary directions on the testing sets of 2D control tasks is reduced by an average of 63.45%. Besides, the visualization of the actual trajectories distribution of the cursor also demonstrates that the decoupling of velocity is capable of yielding accurate cursor control in complex path tracking tasks and significantly improves the control accuracy.
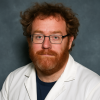
Dr. David Lowemann, M.Sc, Ph.D., is a co-founder of the Institute for the Future of Human Potential, where he leads the charge in pioneering Self-Enhancement Science for the Success of Society. With a keen interest in exploring the untapped potential of the human mind, Dr. Lowemann has dedicated his career to pushing the boundaries of human capabilities and understanding.
Armed with a Master of Science degree and a Ph.D. in his field, Dr. Lowemann has consistently been at the forefront of research and innovation, delving into ways to optimize human performance, cognition, and overall well-being. His work at the Institute revolves around a profound commitment to harnessing cutting-edge science and technology to help individuals lead more fulfilling and intelligent lives.
Dr. Lowemann’s influence extends to the educational platform BetterSmarter.me, where he shares his insights, findings, and personal development strategies with a broader audience. His ongoing mission is shaping the way we perceive and leverage the vast capacities of the human mind, offering invaluable contributions to society’s overall success and collective well-being.