There is a proven correlation between the severity of dementia and reduced brain volumes. Several studies have attempted to use activity data to estimate brain volume as a means of detecting reduction early; however, raw activity data are not directly interpretable and are unstructured, making them challenging to utilize. Furthermore, in the previous research, brain volume estimates were limited to total brain volume and the investigators were unable to detect reductions in specific regions of the brain that are typically used to characterize disease progression. We aimed to evaluate volume prediction of 116 brain regions through activity data obtained combining time-frequency domain- and unsupervised deep learning-based feature extraction methods. We developed a feature extraction model based on unsupervised deep learning using activity data from the National Health and Nutrition Examination Survey (NHANES) dataset (n = 14,482). Then, we applied the model and the time-frequency domain feature extraction method to the activity data of the Biobank Innovations for chronic Cerebrovascular disease With ALZheimer’s disease Study (BICWALZS) datasets (n = 177) to extract activity features. Brain volumes were calculated from the brain magnetic resonance imaging of the BICWALZS dataset and anatomically subdivided into 116 regions. Finally, we fitted linear regression models to estimate each regional volume of the 116 brain areas based on the extracted activity features. Regression models were statistically significant for each region, with an average correlation coefficient of 0.990 ± 0.006. In all brain regions, the correlation was > 0.964. Particularly, regions of the temporal lobe that exhibit characteristic atrophy in the early stages of Alzheimer’s disease showed the highest correlation (0.995). Through a combined deep learning-time-frequency domain feature extraction method, we could extract activity features based solely on the activity dataset, without including clinical variables. The findings of this study indicate the possibility of using activity data for the detection of neurological disorders such as Alzheimer’s disease.
Read Full Article (External Site)
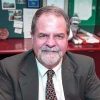
Dr. David Lowemann, M.Sc, Ph.D., is a co-founder of the Institute for the Future of Human Potential, where he leads the charge in pioneering Self-Enhancement Science for the Success of Society. With a keen interest in exploring the untapped potential of the human mind, Dr. Lowemann has dedicated his career to pushing the boundaries of human capabilities and understanding.
Armed with a Master of Science degree and a Ph.D. in his field, Dr. Lowemann has consistently been at the forefront of research and innovation, delving into ways to optimize human performance, cognition, and overall well-being. His work at the Institute revolves around a profound commitment to harnessing cutting-edge science and technology to help individuals lead more fulfilling and intelligent lives.
Dr. Lowemann’s influence extends to the educational platform BetterSmarter.me, where he shares his insights, findings, and personal development strategies with a broader audience. His ongoing mission is shaping the way we perceive and leverage the vast capacities of the human mind, offering invaluable contributions to society’s overall success and collective well-being.