Imagine you’re at a busy restaurant, trying to have a conversation with a friend. The loud noise and chatter make it difficult to focus on their words, but somehow your brain filters out the background noise and allows you to understand them clearly. How does your brain do that? Well, it turns out that our brains have an amazing ability to process sensory information and recognize objects with consistency, even when the input is noisy and incomplete. Scientists have long believed that this is achieved through a hierarchical framework, where different stages of processing build on each other to create invariant object representations. However, recent research challenges this framework and proposes a new principle called Maximal Dependence Capturing (MDC). According to MDC, individual neurons in the brain encode objects by capturing the structural components that contain the most information about them. In other words, each neuron focuses on the most important features of an object to create a consistent representation. This idea has been supported by experimental evidence and computational models. By understanding how our brains process sensory information, we can gain insights into how we perceive the world around us. If you want to dive deeper into this fascinating topic, check out the full article!
Sensory inputs conveying information about the environment are often noisy and incomplete, yet the brain can achieve remarkable consistency in recognizing objects. Presumably, transforming the varying input patterns into invariant object representations is pivotal for this cognitive robustness. In the classic hierarchical representation framework, early stages of sensory processing utilize independent components of environmental stimuli to ensure efficient information transmission. Representations in subsequent stages are based on increasingly complex receptive fields along a hierarchical network. This framework accurately captures the input structures; however, it is challenging to achieve invariance in representing different appearances of objects. Here we assess theoretical and experimental inconsistencies of the current framework. In its place, we propose that individual neurons encode objects by following the principle of maximal dependence capturing (MDC), which compels each neuron to capture the structural components that contain maximal information about specific objects. We implement the proposition in a computational framework incorporating dimension expansion and sparse coding, which achieves consistent representations of object identities under occlusion, corruption, or high noise conditions. The framework neither requires learning the corrupted forms nor comprises deep network layers. Moreover, it explains various receptive field properties of neurons. Thus, MDC provides a unifying principle for sensory processing.
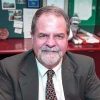
Dr. David Lowemann, M.Sc, Ph.D., is a co-founder of the Institute for the Future of Human Potential, where he leads the charge in pioneering Self-Enhancement Science for the Success of Society. With a keen interest in exploring the untapped potential of the human mind, Dr. Lowemann has dedicated his career to pushing the boundaries of human capabilities and understanding.
Armed with a Master of Science degree and a Ph.D. in his field, Dr. Lowemann has consistently been at the forefront of research and innovation, delving into ways to optimize human performance, cognition, and overall well-being. His work at the Institute revolves around a profound commitment to harnessing cutting-edge science and technology to help individuals lead more fulfilling and intelligent lives.
Dr. Lowemann’s influence extends to the educational platform BetterSmarter.me, where he shares his insights, findings, and personal development strategies with a broader audience. His ongoing mission is shaping the way we perceive and leverage the vast capacities of the human mind, offering invaluable contributions to society’s overall success and collective well-being.