Imagine unraveling the mysteries of Alzheimer’s disease using a treasure map of the brain. That’s exactly what researchers did in this study, where they developed a cutting-edge framework for detecting and analyzing voxel-based features associated with Alzheimer’s disease (AD) using machine learning. By examining 649 voxel-based morphometry (VBM) methods obtained from MRI scans, the researchers created a unique method called Random Survey Support Vector Machines (RS-SVM) to identify important brain regions linked to AD. They then compared the accuracy of different feature detection techniques in three groups: those with early mild cognitive impairment, late mild cognitive impairment, and healthy controls. Remarkably, the RS-SVM method achieved a prediction accuracy of over 90% in distinguishing AD from healthy control subjects. Moreover, the researchers went further and conducted functional analysis of the identified features to uncover their biological significance. The results demonstrated that the identified features are effective for accurately classifying individuals with AD and healthy controls. The RS-SVM framework emerged as the top performer, offering the highest classification accuracy among the tested methods. This exciting research opens up new possibilities for understanding the underlying mechanisms of AD and developing improved diagnostic tools.
Alzheimer’s disease (AD) is a degenerative disease of the central nervous system characterized by memory and cognitive dysfunction, as well as abnormal changes in behavior and personality. The research focused on how machine learning classified AD became a recent hotspot. In this study, we proposed a novel voxel-based feature detection framework for AD. Specifically, using 649 voxel-based morphometry (VBM) methods obtained from MRI in Alzheimer’s Disease Neuroimaging Initiative (ADNI), we proposed a feature detection method according to the Random Survey Support Vector Machines (RS-SVM) and combined the research process based on image-, gene-, and pathway-level analysis for AD prediction. Particularly, we constructed 136, 141, and 113 novel voxel-based features for EMCI (early mild cognitive impairment)-HC (healthy control), LMCI (late mild cognitive impairment)-HC, and AD-HC groups, respectively. We applied linear regression model, least absolute shrinkage and selection operator (Lasso), partial least squares (PLS), SVM, and RS-SVM five methods to test and compare the accuracy of these features in these three groups. The prediction accuracy of the AD-HC group using the RS-SVM method was higher than 90%. In addition, we performed functional analysis of the features to explain the biological significance. The experimental results using five machine learning indicate that the identified features are effective for AD and HC classification, the RS-SVM framework has the best classification accuracy, and our strategy can identify important brain regions for AD.
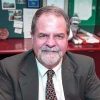
Dr. David Lowemann, M.Sc, Ph.D., is a co-founder of the Institute for the Future of Human Potential, where he leads the charge in pioneering Self-Enhancement Science for the Success of Society. With a keen interest in exploring the untapped potential of the human mind, Dr. Lowemann has dedicated his career to pushing the boundaries of human capabilities and understanding.
Armed with a Master of Science degree and a Ph.D. in his field, Dr. Lowemann has consistently been at the forefront of research and innovation, delving into ways to optimize human performance, cognition, and overall well-being. His work at the Institute revolves around a profound commitment to harnessing cutting-edge science and technology to help individuals lead more fulfilling and intelligent lives.
Dr. Lowemann’s influence extends to the educational platform BetterSmarter.me, where he shares his insights, findings, and personal development strategies with a broader audience. His ongoing mission is shaping the way we perceive and leverage the vast capacities of the human mind, offering invaluable contributions to society’s overall success and collective well-being.