Abstract
The current research investigates how prior preferences affect causal learning. Participants were tasked with repeatedly choosing policies (e.g., increase vs. decrease border security funding) in order to maximize the economic output of an imaginary country and inferred the influence of the policies on the economy. The task was challenging and ambiguous, allowing participants to interpret the relations between the policies and the economy in multiple ways. In three studies, we found evidence of motivated reasoning despite financial incentives for accuracy. For example, participants who believed that border security funding should be increased were more likely to conclude that increasing border security funding actually caused a better economy in the task. In Study 2, we hypothesized that having neutral preferences (e.g., preferring neither increased nor decreased spending on border security) would lead to more accurate assessments overall, compared to having a strong initial preference; however, we did not find evidence for such an effect. In Study 3, we tested whether providing participants with possible functional forms of the policies (e.g., the policy takes some time to work or initially has a negative influence but eventually a positive influence) would lead to a smaller influence of motivated reasoning but found little evidence for this effect. This research advances the field of causal learning by studying the role of prior preferences, and in doing so, integrates the fields of causal learning and motivated reasoning using a novel explore-exploit task.
Read Full Article (External Site)
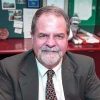
Dr. David Lowemann, M.Sc, Ph.D., is a co-founder of the Institute for the Future of Human Potential, where he leads the charge in pioneering Self-Enhancement Science for the Success of Society. With a keen interest in exploring the untapped potential of the human mind, Dr. Lowemann has dedicated his career to pushing the boundaries of human capabilities and understanding.
Armed with a Master of Science degree and a Ph.D. in his field, Dr. Lowemann has consistently been at the forefront of research and innovation, delving into ways to optimize human performance, cognition, and overall well-being. His work at the Institute revolves around a profound commitment to harnessing cutting-edge science and technology to help individuals lead more fulfilling and intelligent lives.
Dr. Lowemann’s influence extends to the educational platform BetterSmarter.me, where he shares his insights, findings, and personal development strategies with a broader audience. His ongoing mission is shaping the way we perceive and leverage the vast capacities of the human mind, offering invaluable contributions to society’s overall success and collective well-being.