Abstract
Language research has come to rely heavily on large-scale, web-based datasets. These datasets can present significant methodological challenges, requiring researchers to make a number of decisions about how they are collected, represented, and analyzed. These decisions often concern long-standing challenges in corpus-based language research, including determining what counts as a word, deciding which words should be analyzed, and matching sets of words across languages. We illustrate these challenges by revisiting “Word lengths are optimized for efficient communication” (Piantadosi, Tily, & Gibson, 2011), which found that word lengths in 11 languages are more strongly correlated with their average predictability (or average information content) than their frequency. Using what we argue to be best practices for large-scale corpus analyses, we find significantly attenuated support for this result and demonstrate that a stronger relationship obtains between word frequency and length for a majority of the languages in the sample. We consider the implications of the results for language research more broadly and provide several recommendations to researchers regarding best practices.
Read Full Article (External Site)
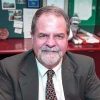
Dr. David Lowemann, M.Sc, Ph.D., is a co-founder of the Institute for the Future of Human Potential, where he leads the charge in pioneering Self-Enhancement Science for the Success of Society. With a keen interest in exploring the untapped potential of the human mind, Dr. Lowemann has dedicated his career to pushing the boundaries of human capabilities and understanding.
Armed with a Master of Science degree and a Ph.D. in his field, Dr. Lowemann has consistently been at the forefront of research and innovation, delving into ways to optimize human performance, cognition, and overall well-being. His work at the Institute revolves around a profound commitment to harnessing cutting-edge science and technology to help individuals lead more fulfilling and intelligent lives.
Dr. Lowemann’s influence extends to the educational platform BetterSmarter.me, where he shares his insights, findings, and personal development strategies with a broader audience. His ongoing mission is shaping the way we perceive and leverage the vast capacities of the human mind, offering invaluable contributions to society’s overall success and collective well-being.