Over the last few decades, electroencephalogram (EEG) has become one of the most vital tools used by physicians to diagnose several neurological disorders of the human brain and, in particular, to detect seizures. Because of its peculiar nature, the consequent impact of epileptic seizures on the quality of life of patients made the precise diagnosis of epilepsy extremely essential. Therefore, this article proposes a novel deep-learning approach for detecting seizures in pediatric patients based on the classification of raw multichannel EEG signal recordings that are minimally pre-processed. The new approach takes advantage of the automatic feature learning capabilities of a two-dimensional deep convolution autoencoder (2D-DCAE) linked to a neural network-based classifier to form a unified system that is trained in a supervised way to achieve the best classification accuracy between the ictal and interictal brain state signals. For testing and evaluating our approach, two models were designed and assessed using three different EEG data segment lengths and a 10-fold cross-validation scheme. Based on five evaluation metrics, the best performing model was a supervised deep convolutional autoencoder (SDCAE) model that uses a bidirectional long short-term memory (Bi-LSTM) – based classifier, and EEG segment length of 4 s. Using the public dataset collected from the Children’s Hospital Boston (CHB) and the Massachusetts Institute of Technology (MIT), this model has obtained 98.79 ± 0.53% accuracy, 98.72 ± 0.77% sensitivity, 98.86 ± 0.53% specificity, 98.86 ± 0.53% precision, and an F1-score of 98.79 ± 0.53%, respectively. Based on these results, our new approach was able to present one of the most effective seizure detection methods compared to other existing state-of-the-art methods applied to the same dataset.
Read Full Article (External Site)
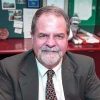
Dr. David Lowemann, M.Sc, Ph.D., is a co-founder of the Institute for the Future of Human Potential, where he leads the charge in pioneering Self-Enhancement Science for the Success of Society. With a keen interest in exploring the untapped potential of the human mind, Dr. Lowemann has dedicated his career to pushing the boundaries of human capabilities and understanding.
Armed with a Master of Science degree and a Ph.D. in his field, Dr. Lowemann has consistently been at the forefront of research and innovation, delving into ways to optimize human performance, cognition, and overall well-being. His work at the Institute revolves around a profound commitment to harnessing cutting-edge science and technology to help individuals lead more fulfilling and intelligent lives.
Dr. Lowemann’s influence extends to the educational platform BetterSmarter.me, where he shares his insights, findings, and personal development strategies with a broader audience. His ongoing mission is shaping the way we perceive and leverage the vast capacities of the human mind, offering invaluable contributions to society’s overall success and collective well-being.