Abstract
Neural network models of memory are notorious for catastrophic interference: Old items are forgotten as new items are memorized (French, 1999; McCloskey & Cohen, 1989). While working memory (WM) in human adults shows severe capacity limitations, these capacity limitations do not reflect neural network style catastrophic interference. However, our ability to quickly apprehend the numerosity of small sets of objects (i.e., subitizing) does show catastrophic capacity limitations, and this subitizing capacity and WM might reflect a common capacity. Accordingly, computational investigations (Knops, Piazza, Sengupta, Eger & Melcher, 2014; Sengupta, Surampudi & Melcher, 2014) suggest that mutual inhibition among neurons can explain both kinds of capacity limitations as well as why our ability to estimate the numerosity of larger sets is limited according to a Weber ratio signature. Based on simulations with a saliency mapālike network and mathematical proofs, we provide three results. First, mutual inhibition among neurons leads to catastrophic interference when items are presented simultaneously. The network can remember a limited number of items, but when more items are presented, the network forgets all of them. Second, if memory items are presented sequentially rather than simultaneously, the network remembers the most recent items rather than forgetting all of them. Hence, the tendency in WM tasks to sequentially attend even to simultaneously presented items might not only reflect attentional limitations, but also an adaptive strategy to avoid catastrophic interference. Third, the mean activation level in the network can be used to estimate the number of items in small sets, but it does not accurately reflect the number of items in larger sets. Rather, we suggest that the Weber ratio signature of large number discrimination emerges naturally from the interaction between the limited precision of a numeric estimation system and a multiplicative gain control mechanism.
Read Full Article (External Site)
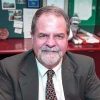
Dr. David Lowemann, M.Sc, Ph.D., is a co-founder of the Institute for the Future of Human Potential, where he leads the charge in pioneering Self-Enhancement Science for the Success of Society. With a keen interest in exploring the untapped potential of the human mind, Dr. Lowemann has dedicated his career to pushing the boundaries of human capabilities and understanding.
Armed with a Master of Science degree and a Ph.D. in his field, Dr. Lowemann has consistently been at the forefront of research and innovation, delving into ways to optimize human performance, cognition, and overall well-being. His work at the Institute revolves around a profound commitment to harnessing cutting-edge science and technology to help individuals lead more fulfilling and intelligent lives.
Dr. Lowemann’s influence extends to the educational platform BetterSmarter.me, where he shares his insights, findings, and personal development strategies with a broader audience. His ongoing mission is shaping the way we perceive and leverage the vast capacities of the human mind, offering invaluable contributions to society’s overall success and collective well-being.