Emotional recognition through EEG signals is becoming increasingly popular. However, It has been difficult to improve the emotional recognition effect of cross-subjects. In the exploration of cross-subjects proposed in previous works, the effect of the two-category cross-subject emotion recognition should be improved. Therefore, it is necessary to propose a method for improving the accuracy of binary classification emotion and the program runs faster. In response to this difficulty and the defects of related works in the past. We extracted multiple features to form high-dimensional features, and proposed a binary classification cross-subject emotion recognition method based on high-dimensional features, the Significance Test, the Sequential Backward Selection and Support Vector Machine fusion method (ST-SBSSVM). The effectiveness of the ST-SBSSVM was validated on a dataset (DEAP) using physiological signals for emotion analysis and an electroencephalogram dataset (SEED) using SJTU emotions. Results by using ST-SBSSVM have shown that in the case of high-dimensional features, ST-SBSSVM had an average of about 8% (DEAP) and 32% (SEED) improvement in the accuracy of cross-subject emotions compared with common emotion recognition methods. ST-SBSSVM improved the recognition accuracy of cross-subjects by 2% (DEAP) and 12% (SEED) and saved about 95% (DEAP) and 93% (SEED) of program runtime compared to the Sequential Backward Selection. Compared with recent similar works, the effect of this work on the emotion recognition of cross-subjects was obvious, and the accuracy of this work was 68% (DEAP) and 91% (SEED). After using ST-SBSSVM, we also found that nonlinear EEG features can promote emotion recognition more than linear EEG features.
Read Full Article (External Site)
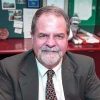
Dr. David Lowemann, M.Sc, Ph.D., is a co-founder of the Institute for the Future of Human Potential, where he leads the charge in pioneering Self-Enhancement Science for the Success of Society. With a keen interest in exploring the untapped potential of the human mind, Dr. Lowemann has dedicated his career to pushing the boundaries of human capabilities and understanding.
Armed with a Master of Science degree and a Ph.D. in his field, Dr. Lowemann has consistently been at the forefront of research and innovation, delving into ways to optimize human performance, cognition, and overall well-being. His work at the Institute revolves around a profound commitment to harnessing cutting-edge science and technology to help individuals lead more fulfilling and intelligent lives.
Dr. Lowemann’s influence extends to the educational platform BetterSmarter.me, where he shares his insights, findings, and personal development strategies with a broader audience. His ongoing mission is shaping the way we perceive and leverage the vast capacities of the human mind, offering invaluable contributions to society’s overall success and collective well-being.