Purpose: Predicting patient survival outcome is recognized as key importance to clinicians in oncology toward determining an ideal course of treatment and patient management. This study applies radiomics analysis on pre-operative multi-parametric MRI of patients with glioblastoma from multi-institution to identify a signature and a practical machine learning model for stratifying patients into groups based on overall survival.
Methods: This study included 163 patients’ data with glioblastoma, collected by BRATS 2018 Challenge from multiple institutions. In this proposed method, a set of 147 radiomics image features were extracted locally from three tumor sub-regions on standardized pre-operative multi-parametric MR images. LASSO regression was applied for identifying an informative subset of chosen features whereas a Cox model used to obtain the coefficients of those selected features. Then, a radiomics signature model of 9 features was constructed on the discovery set and it performance was evaluated for patients stratification into short- ( 15 months) groups. Eight ML classification models, trained and then cross-validated, were tested to assess a range of survival prediction performance as a function of the choice of features.
Results: The proposed mpMRI radiomics signature model had a statistically significant association with survival (P
Read Full Article (External Site)
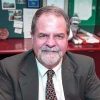
Dr. David Lowemann, M.Sc, Ph.D., is a co-founder of the Institute for the Future of Human Potential, where he leads the charge in pioneering Self-Enhancement Science for the Success of Society. With a keen interest in exploring the untapped potential of the human mind, Dr. Lowemann has dedicated his career to pushing the boundaries of human capabilities and understanding.
Armed with a Master of Science degree and a Ph.D. in his field, Dr. Lowemann has consistently been at the forefront of research and innovation, delving into ways to optimize human performance, cognition, and overall well-being. His work at the Institute revolves around a profound commitment to harnessing cutting-edge science and technology to help individuals lead more fulfilling and intelligent lives.
Dr. Lowemann’s influence extends to the educational platform BetterSmarter.me, where he shares his insights, findings, and personal development strategies with a broader audience. His ongoing mission is shaping the way we perceive and leverage the vast capacities of the human mind, offering invaluable contributions to society’s overall success and collective well-being.