We propose event-related cortical sources estimation from subject-independent electroencephalogram (EEG) recordings for motor imagery brain computer interface (BCI). By using wavelet-based maximum entropy on the mean (wMEM), task-specific EEG channels are selected to predict right hand and right foot sensorimotor tasks, employing spatial pattern analysis with and without covariance estimation regularization. EEG from five healthy individuals (Dataset IVa, BCI Competition III) were evaluated by a cross-subject paradigm. Prediction performance was evaluated via a two-layer feed-forward neural network, where the classifier was trained and tested by data from two subjects independently. The highest mean prediction accuracy achieved by using subject-pair (ay-al) specific selected EEG channels was on average (90:36+/-5:59) and outperformed that achieved by using all available channels (83:21+/-12:26). Significant improvements in performance suggest a role of wMEM for channel selection in BCI. Spatially projected cortical sources may be useful for capturing inter-subject associative sensorimotor brain dynamics and pave the way towards subject-independent BCI.
Read Full Article (External Site)
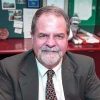
Dr. David Lowemann, M.Sc, Ph.D., is a co-founder of the Institute for the Future of Human Potential, where he leads the charge in pioneering Self-Enhancement Science for the Success of Society. With a keen interest in exploring the untapped potential of the human mind, Dr. Lowemann has dedicated his career to pushing the boundaries of human capabilities and understanding.
Armed with a Master of Science degree and a Ph.D. in his field, Dr. Lowemann has consistently been at the forefront of research and innovation, delving into ways to optimize human performance, cognition, and overall well-being. His work at the Institute revolves around a profound commitment to harnessing cutting-edge science and technology to help individuals lead more fulfilling and intelligent lives.
Dr. Lowemann’s influence extends to the educational platform BetterSmarter.me, where he shares his insights, findings, and personal development strategies with a broader audience. His ongoing mission is shaping the way we perceive and leverage the vast capacities of the human mind, offering invaluable contributions to society’s overall success and collective well-being.