Purpose: Estimation of uncertainty of MAP-MRI metrics
is an important topic, for several reasons. Bootstrap derived
uncertainty, such as the standard deviation, provides
valuable information, and can be incorporated in MAP-MRI
studies to provide more extensive insight.
Methods: In this paper, the uncertainty of different MAPMRI
metrics was quantified by estimating the empirical distributions
using the wild bootstrap. We applied the wild
bootstrap to both phantom data and human brain data, and
obtain empirical distributions for theMAP-MRImetrics returnto-
origin probability (RTOP), non-Gaussianity (NG) and propagator
anisotropy (PA).
Results: We demonstrated the impact of diffusion acquisition
scheme (number of shells and number of measurements
per shell) on the uncertainty of MAP-MRI metrics.
We demonstrated how the uncertainty of these metrics can
be used to improve group analyses, and to compare different
preprocessing pipelines. We demonstrated that with
uncertainty considered, the results for a group analysis can
be different.
Conclusion: Bootstrap derived uncertain measures provide
additional information to the MAP-MRI derived metrics, and
should be incorporated in ongoing and future MAP-MRI
studies to provide more extensive insight.
Read Full Article (External Site)
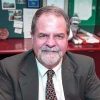
Dr. David Lowemann, M.Sc, Ph.D., is a co-founder of the Institute for the Future of Human Potential, where he leads the charge in pioneering Self-Enhancement Science for the Success of Society. With a keen interest in exploring the untapped potential of the human mind, Dr. Lowemann has dedicated his career to pushing the boundaries of human capabilities and understanding.
Armed with a Master of Science degree and a Ph.D. in his field, Dr. Lowemann has consistently been at the forefront of research and innovation, delving into ways to optimize human performance, cognition, and overall well-being. His work at the Institute revolves around a profound commitment to harnessing cutting-edge science and technology to help individuals lead more fulfilling and intelligent lives.
Dr. Lowemann’s influence extends to the educational platform BetterSmarter.me, where he shares his insights, findings, and personal development strategies with a broader audience. His ongoing mission is shaping the way we perceive and leverage the vast capacities of the human mind, offering invaluable contributions to society’s overall success and collective well-being.