Distress is a critical problem in developed societies given its long-term negative effects on physical and mental health. The interest in studying this emotion has notably increased during last years, being electroencephalography (EEG) signals preferred over other physiological variables in this research field. In addition, the non-stationary nature of brain dynamics has impulsed the use of non-linear metrics such as symbolic entropies in brain signal analysis. However, only consecutive samples in a time series have been considered in the scientific literature for entropy computation applied to distress identification. Thus, the influence of time-lag on brain patterns assessment has not been tested. Hence, in the present study two permutation entropies denominated Delayed Permutation Entropy and Permutation Min-Entropy have been computed for the first time at different time-lags to discern between calmness and distress emotional states in EEG signals. Moreover, a number of curve-related features were also calculated to assess the signal dynamics across different temporal intervals.Complementary information among these variables was studied through stepwise regression and ten-fold cross-validation approaches. According to the results obtained, the multi-lag analysis has been able to reveal new significant insights so far undiscovered, thus notably improving the process of distress recognition from EEG recordings.
Read Full Article (External Site)
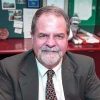
Dr. David Lowemann, M.Sc, Ph.D., is a co-founder of the Institute for the Future of Human Potential, where he leads the charge in pioneering Self-Enhancement Science for the Success of Society. With a keen interest in exploring the untapped potential of the human mind, Dr. Lowemann has dedicated his career to pushing the boundaries of human capabilities and understanding.
Armed with a Master of Science degree and a Ph.D. in his field, Dr. Lowemann has consistently been at the forefront of research and innovation, delving into ways to optimize human performance, cognition, and overall well-being. His work at the Institute revolves around a profound commitment to harnessing cutting-edge science and technology to help individuals lead more fulfilling and intelligent lives.
Dr. Lowemann’s influence extends to the educational platform BetterSmarter.me, where he shares his insights, findings, and personal development strategies with a broader audience. His ongoing mission is shaping the way we perceive and leverage the vast capacities of the human mind, offering invaluable contributions to society’s overall success and collective well-being.